Scientists and students at the University of Portsmouth have recently published results of a research project which placed these unprecedently large fires in a wider context. Satellite imagery and image classification techniques within ERDAS IMAGINE were used to explore the history and severity of wildfires in Greenland since 1995. The approach focused on four study areas in western Greenland and acquired Landsat 4/5 TM, Landsat 7 ETM+, Landsat 8 OLI and Sentinel-2A multispectral satellite images for all available years between 1995 and 2020 from the USGS Earth Explorer archive
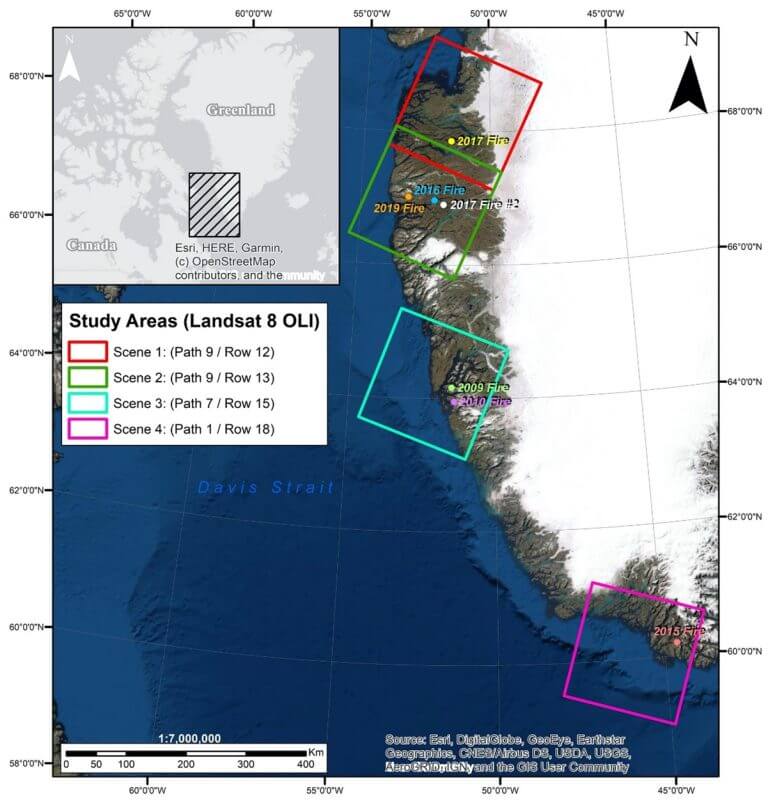
Where possible, images for each year were obtained for early June/July (pre-fire image) and at the end of August/September (post- fire image) in order to capture the likely timing of fires over the summer. To identify fires, the normalised burn ratio (NBR) remote sensing index was used.
The NBR compares the near infrared (NIR) and shortwave infrared (SWIR) bands in the formula NBR = (NIR – SWIR) / (NIR + SWIR). Burned areas have negative NBR values closer to -1 (NBR < SWIR) whereas unburned vegetation have strongly positive NBR values closer to +1 (NIR > SWIR). This approach allowed the University of Portsmouth researchers to identify burn scars for additional fires in western Greenland in 2009, 2010, 2015, 2016 and 2017.
“THE NORMALISED BURN RATIO COMPARES THE NEAR INFRARED (NIR) AND SHORTWAVE INFRARED (SWIR) BANDS...”
The burn severity for the fires was assessed by producing differenced NBR (dNBR) images (subtracts the post-fire NBR image from the pre-fire NBR image) and relative dNBR (RdNBR) images (removes any pre-fire vegetation bias by dividing dNBR by the square root of the pre-fire NBR). A spatial model downloaded from the Hexagon Geospatial Support website was used to do this. The RdNBR images were used to produce burned area reflectance classification (BARC) maps for the identified fires. These allow the spatial variation in burn severity within the burned area to be assessed.
Finally, post-fire vegetation recovery was explored using a series of normalised differenced vegetation index (NDVI) images NDVI = (NIR– RED) / (NIR + RED). For the 2017 fire, these images showed that vegetation recovery was well underway three years after the fire.
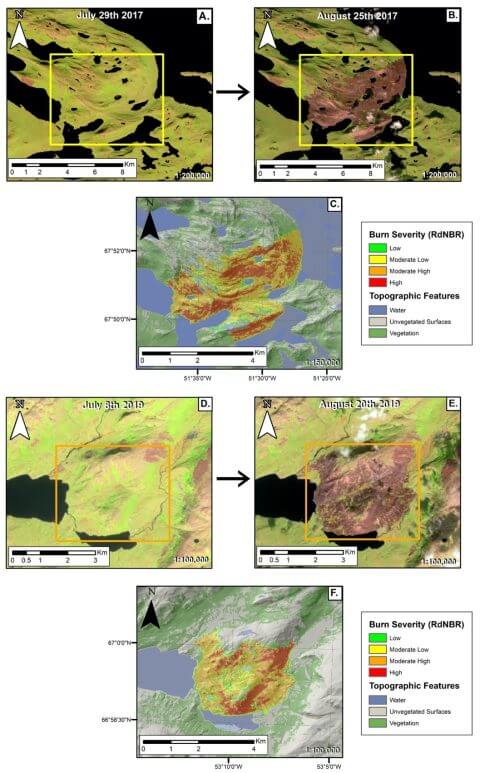
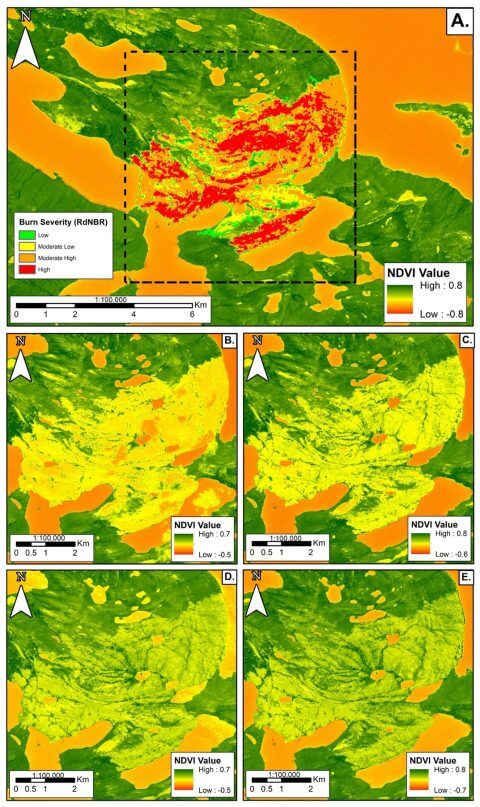
A. Sentinel-2 false colour pre-fire image of the 2017 fire (image date: 29/07/17). Yellow box shows fire
B. Sentinel-2 false colour post-fire image of the 2017 fire (image date: 25/08/17).
C.2017 fire burn severity overlain on an unsupervised landcover
D.Sentinel-2 false colour pre-fire image of the 2019 fire (image date: 08/07/19). Orange box shows fire
E. Sentinel-2 false colour post-fire image of the 2019 fire (image date: 20/08/19).
F.2019 fire burn severity overlain on an unsupervised landcover
Post-fire NDVI images for the 2017 fire. Darker green areas correspond to unburned vegetation, lighter green and yellow areas identify bare ground and burned vegetation.
A. 2017 burn severity overlain on pre-fire NDVI image (image date: 29/07/17).
B. Immediately post-fire (image date: 25/08/17).
C. One year post-fire (image date: 20/08/18).
D..Two years post-fire (image date: 18/08/19).
E. Three years post-fire (image date: 03/07/20).
Results...
The research shows that fires are more common in western Greenland than previously thought, although it is interesting to note that fires prior to 2009 were not detectable using this remote sensing approach. More details on the history of fires in western Greenland can be found in the International Journal of Wildland Fire.
“Fires are more common in western Greenland than previously thought…”
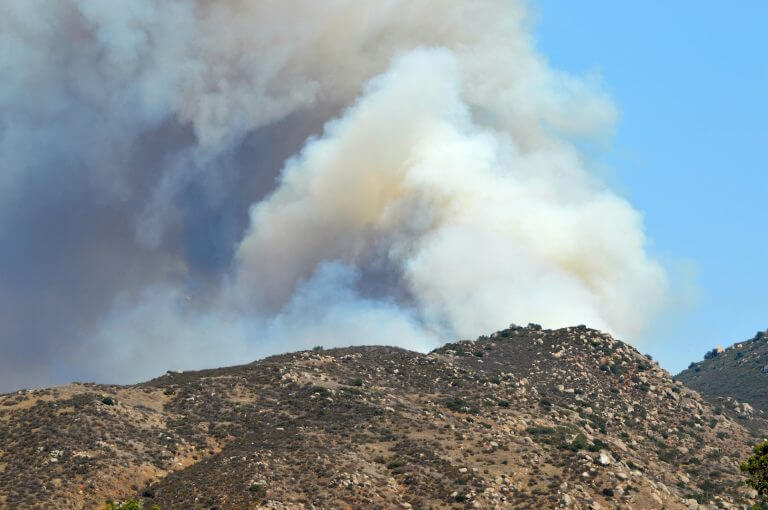
The remote sensing aspects of this work were completed by Ben Gosden as part of his postgraduate research project on the Geographical Information Systems MSc at the University of Portsmouth
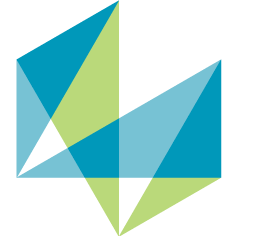
