The population of African elephants has been dramatically declining over the last decade, with around only 415,000 African Savannah Elephants left in the wild today. Herds of the world’s largest land mammal roam across the grassy plains and bushlands of eastern and southern Africa; more specifically found in Botswana, Tanzania, Zimbabwe, Kenya, Namibia, Mozambique and South Africa. African Elephants are the most heavily poached animal in the world, in demand for their ivory tusks, which fetch extremely high prices on the black market. They are also faced with habitat loss, as the human population grows and natural environments are increasingly lost to housing and agricultural land uses. Increasing proximity to human populations can also cause issues when elephants eat or destroy crops, sometimes leading to retaliatory killing of the animals.
Isla Duporge, PhD Researcher from the Wildlife Conservation Research Unit, University of Oxford recently led a team to remotely survey African elephants using a combination satellite imagery and deep learning techniques to automatically detect the animals. The initial research focused on Addo Elephant Park, South Africa – the third largest game park in the country – because of its large concentration of elephants and varied terrain.
"THE ALGORITHMS... DEMONSTRATED HIGH LEVELS OF ACCURACY, OUTPERFORMING CONVENTIONAL METHODS..."
Being able to accurately observe and monitor the highly endangered elephant population is crucial for their conservation, as well as the maintenance of suitable habitats for other species that rely on them and their impact upon the landscape. Currently, the most commonly used method of monitoring is by conducting surveys with manned aircraft, but this is relatively costly and sometimes unreliable, leading to inaccurate data as a result. Tracking wild animals through the use of satellite remote sensing had only previously been completed in homogeneous landscapes, such as monitoring whales in the oceans. African Elephants wander amongst both grasslands and forests, so to collate accurate data it was important to develop a robust methodology to track them across a range of different landscapes
Acquiring satellite data from the WorldView-3 and WorldView-4 satellites meant the team could obtain images with a 32cm spatial resolution. To analyse this data, ERDAS IMAGINE was the ideal solution, including a number of built-in features targeted at optimising information extraction from Very-High Resolution (VHR) imagery such as WorldView-3 and WorldView-4.
Once the images had been pan-sharpened using the Gram-Schmidt algorithm, over 1,000 elephants were labelled and used to develop a deep learning algorithm which enabled automatic detection of individual elephants across large areas. In comparison with aircraft surveys, the use of satellite imagery provides a synoptic view of large land areas in just a few images. This approach significantly reduced the error levels in counting elephant numbers, and with easy access to an abundance of up-to-date imagery, repeat surveys could be completed in a short amount of time.
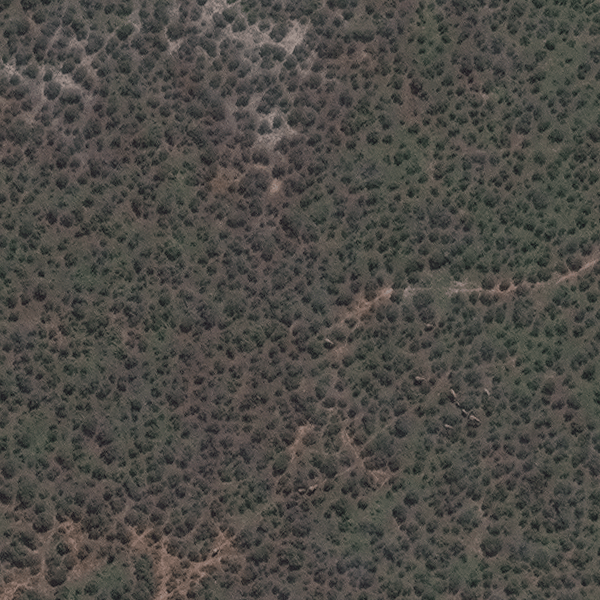
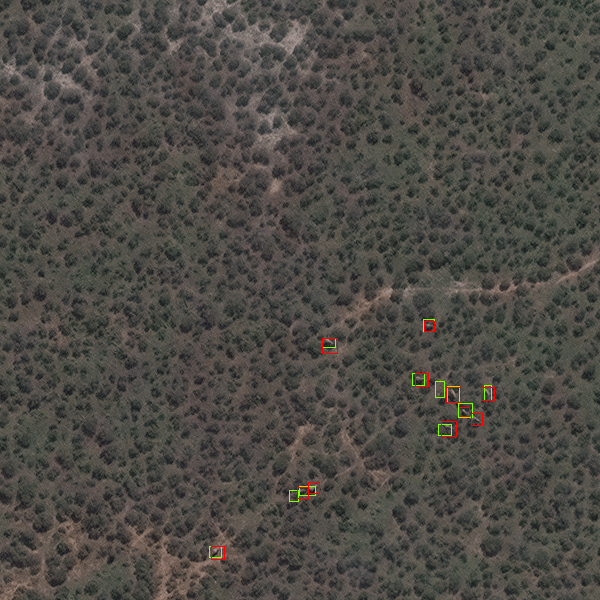
Extracts from World-View – 18th October 2008. Once processed through a deep learning algorithm, detected elephants can be seen amongst heavy vegetation.
The algorithm was tested again in Kenya, where it successfully detected large numbers of elephants, as well as their calves, which hadn’t been explored in the previous part of the study.
There are many animal species at risk of extinction across the globe and the success of this study illustrates the potential for it to be applied more widely. In particular, it is a highly suitable method for use in trans-frontier or conflict areas where manned aircraft flights may not be permitted or extremely dangerous. The key issue faced by previous surveys of animal populations is the ability to systematically cover large areas, especially if those areas are inaccessible, in difficult terrain or in conflict zones where physical access is challenging. Through the use of remote sensing, varied habitats can be studied without the need for direct human interaction on the ground, leaving the animals and their habitats undisturbed and physical risk to the human observers is eliminated.
The algorithms developed to identify and count elephants demonstrated high levels of accuracy, outperforming conventional methods within heterogeneous landscapes. Shown to be both a cost-effective and highly accurate monitoring technique, remote sensing could have the capabilities to monitor wildlife across various landscapes, improving our ability to protect critically endangered species whilst reducing human risk, resource costs and time restraints.